- December 27, 2023
- Category: What's New
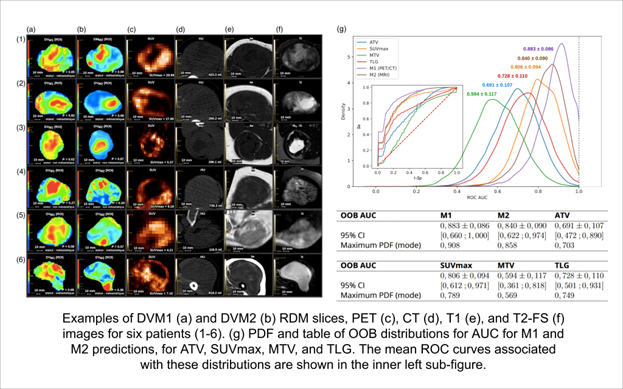
EFOMP NEWSLETTER WINTER ISSUE 12/2023 | COMPANY MEMBER ΑRTICLE
Research and innovation have been two important pillars of DOSIsoft since its creation. In recent years, Artificial Intelligence (AI) has been introduced into the medical domain, especially in radiation oncology and nuclear medicine. The application of Machine Learning (ML) and Deep Learning (DL) techniques is growing considerably to gain a deeper understanding of patient data, offer powerful radiotherapy patient safety, and contribute to more personalized cancer patient care.
Guided by this trend, DOSIsoft initiated two medical physics thesis works, successfully completed in November 2023. The research outcomes show that AI models could be a promising alternative algorithmic approach to allow automation and optimisation of the workflow in radiotherapy, such as auto-segmentation, diagnosis, treatment planning, and the external radiotherapy QA process.
Lucas DAL BOSCO introduces a new dosimetry tool by Artificial Neural Networks for Patient-Specific Quality Assurance in External Beam Radiotherapy – In collaboration with Dr. Marie-Véronique Le Lann (CNRS LAAS DISCO Laboratory, Toulouse, France).
In this work, two CNNs were developed for EPID-based non-transit dosimetry. The first model was trained to convert acquired gray-scale portal images into absolute dose distributions. The second model was trained to predict absolute dose distributions from treatment planning data. For both computational engines, a specific architecture based on the U-net model coupled with a non-trainable neural layer called True Dose Modulation (TDM) and a two-step training method were developed. The calculated dose distributions show a very good agreement with those of current analytical models. GAI scores higher than 97.40% and 98.09% (criteria of 2% / 2 mm > 10% Dmax of the ϒ-index) were obtained by the conversion CNN and the prediction CNN for all clinical control beams, respectively.
This work also aimed to propose a formalism and a methodology for applying CNNs to IVD. A reference system based on a commercially available solution was developed with the purpose of producing the data needed to develop DL models applied to transit dosimetry. A first CNN was developed to predict the scattered dose from the attenuator in transit conditions. The results obtained show a good reconstruction of the relative contribution of the scattered dose. An average GAI of 90.66% was obtained with the clinical control beams.
To conclude, this work shows that DL models could be a promising alternative algorithmic approach for EPID-based dosimetry applications.
Thibault ESCOBAR introducing radiomic models with machine learning approaches for planning and monitoring cancer treatments using multi-parametric PET/CT/MRI imaging – In collaboration with Dr Irène Buvat Laboratoire d’Imagerie Translationnelle en Oncologie – U1023 Inserm/Institut Curie, France
This thesis explores the growing role of digital sciences in healthcare, particularly in the field of medical imaging for cancer. The use of different medical imaging techniques, such as computed tomography (CT), positron emission tomography (PET) and magnetic resonance imaging (MRI), plays an essential role for the diagnosis, treatment planning and follow-up of cancer patients.
The adoption of radiomics and machine learning approaches in clinical practice seems promising, but remains limited today, partly due to the lack of interpretability of the associated models.
This thesis addresses this challenge by developing new methods integrating modeling, visualization, and model mapping, with an emphasis on simplicity. The aim is to provide clinicians and researchers with an in-depth understanding of the information underlying the decisions suggested by models, in order to facilitate their adoption and use in clinical decision-making. This research contributes to the development of robust, reliable, and clinically relevant models and biomarkers, to improve the management of cancer patients.
About DOSIsoft – Founded in 2002, DOSIsoft designs, develops & delivers patient-specific imaging & dosimetry software solutions in Radiation Oncology & Nuclear Medicine to improve cancer patient safety & treatment quality. 20 years of innovation and R&D investments have led to world leading software used in over 600 hospital centres in 60 countries. Spin-off between Gustave Roussy and Institut Curie, DOSIsoft constantly innovates in partnership with the major cancer institutes and research centres in the world. It is now recognized as a key player in the dosimetry market. www.dosisoft.com